-
Potatoes (Solanum tuberosum L.) are among the most widely consumed non-grain foods worldwide, becoming a staple crop in many countries due to their importance in the food industry supply chain[1]. Global agriculture is facing shortages of skilled labor, climate impacts, and population growth, which pose significant challenges to modern agriculture. Potatoes play a vital role in meeting the nutritional needs of the population and are the fourth most widely cultivated crop in the world after wheat, rice, and maize[2,3]. Globally, potato production has increased by 11% over the past two decades, from 322 million tons in 2000 to 354 million tons in 2020. Asia produced 49.7%, Europe 30%, and North and South America 12.5% of the total. In 2020, China produced 78.2 million tons, India 51.3 million tons, and Ukraine 20.8 million tons, accounting for more than 40% of the world's total potato production. Although potato production fell by 17% from about 19.9 million hectares in 2000 to about 16.4 million hectares in 2020, potato yields increased by 34%, from about 16.2 t/ha in 2000 to about 21.8 t/ha in 2020[4]. Potato harvesting is one of the most influential stages in the potato production line for yield losses[5]. Potato harvesting involves the separation and collection of tubers from the soil, and losses can occur due to damage or leaving potatoes in the field. There is no single approach that can be applied effectively across farms, geographic environments, or soil types. The design of a mechanical harvester is influenced by environmental conditions, and regional factors and available harvesting methods play a major role in potato production[6]. There are many methods of harvesting potatoes, with manual harvesting being the simplest and can be done using simple tools such as a pick or fork, or even without equipment. However, manual harvesting is time-consuming and labor-intensive[7]. To address these challenges, animal-powered harvesting machines, such as traditional plows, have been developed, and these two methods are still popular in many regions around the world, although the use of animals can sometimes be subject to neglect or injury[8,9]. The complexity of the harvesting process increases with the introduction of fully mechanical and semi-mechanical harvesters. Fully mechanical harvesters are characterized by their ability to collect potatoes directly into a cart or bunker during the harvesting process, eliminating the need for manual labor to collect potatoes from the field after harvesting is complete. These harvesters are a significant improvement over traditional harvesting methods, contributing to reduced harvesting time, lower costs, and reduced crop losses[10−12].
Modern technologies such as automation and artificial intelligence are improving the efficiency, accuracy, and sustainability of potato harvesting operations, although, to date, there are no working prototypes in the academic literature or industry[13]. After harvesting, potatoes encounter many impurities, such as lumps and stones that are similar in size to potatoes, which makes them difficult to separate. Therefore, it is necessary to use accurate sorting systems that can improve sorting quality, such as automated systems based on machine vision[14]. Computer vision systems have been used in agriculture for many years, including sorting fruits[15], grains[16], and pathogen detection[17]. By analyzing data and images, the physical and mechanical characteristics of potatoes can be sorted. This review aims to clarify the basic differences between manual and mechanical potato harvesting, facilitating an understanding of the advantages and disadvantages of each method. It also reviews the challenges facing potato harvesting machinery design, including potato and soil characteristics, as well as the performance of modern harvesting machinery and how to improve its efficiency. This review also discusses innovations in this field that contribute to increasing productivity and reducing waste, and highlights the role of artificial intelligence in improving the post-harvest potato sorting processes, which enhances the quality of the final product. In contrast, the review is limited to focusing on the differences between manual and mechanical harvesting without addressing costs or the impact of climatic and economic conditions.
-
Potato harvesting in the production system is a vital and one of the most expensive stages in the production process[18]. The harvesting process is usually carried out using manual, semi-mechanized, or fully mechanized methods[19]. In the case of semi-mechanized harvesting, excavators attached to tractors are used, which break up the furrows and expose the tubers, while the tubers are collected manually by laborers (Fig. 1).
Figure 1.
Semi-mechanical harvesting process. (a) Potato digger. (b) Manual potato collecting[20].
In developed countries, self-propelled harvesters are used for potato harvesting (Fig. 2). The trend toward full mechanization of the harvesting process is linked to the availability and costs of labor. These harvesters cut furrows and collect potatoes in two or more rows, then direct them to transport trucks. These machines are characterized by their large size, which requires long rows to reduce the need for repeated maneuvers, thus avoiding time wasting that could negatively affect the operational efficiency of the machine[21]. Gomes[19] also points out that mechanical harvesting of potatoes represents a major step towards improving production in agricultural areas, as it contributes to increasing the production area, and helps to remove tubers more quickly from the ground when they are free from the risk of pests and diseases, which enhances adherence to delivery dates. However, deciding to invest in this equipment requires a careful assessment of the risks, as purchasing harvesters represents a major investment that is only justified if it has a significant impact on the profitability of the activity[22]. The selection of agricultural machinery is a complex process, with many variables to consider, and the choice of the right equipment for the farm is one of the most important stages of production[23]. Specialized potato harvesters have begun to exchange experiences with imported potato harvesters, which has led to an increased demand for information on the real opportunities for improvement that mechanization of harvesting can provide[19].
Figure 2.
Mechanical potato harvester[20].
The study by Da Cunha et al.[20] indicates that the total cost of mechanical harvesting was 49.03% lower than that of semi-mechanical harvesting. The operational efficiency of mechanical harvesting was significantly superior to semi-mechanical harvesting, with one harvester being equivalent in capacity to the work of 23 workers in manual harvesting. Mechanical harvesting recorded losses of 2.35% in terms of area productivity, while semi-mechanical harvesting had higher losses, reaching 6.32%.
-
Understanding the diverse characteristics of potatoes can contribute to the development of more efficient harvesters. Considering these characteristics while designing mechanical harvesters and post-harvest equipment can enhance productivity and reduce losses. Ahangarnezhad et al.[24] classified the properties of Agria potato into two types: physical (geometric and arithmetic average diameters, mass, and volume), and mechanical (elastic modulus, deformation energy, and breaking strength). These are important when designing potato sorting and packing machines, as they help reduce losses during transportation and separate potatoes from other materials during the harvesting process. These mechanical properties can be determined by uniaxial compression testing. Absorption energy is important, as higher energy absorption leads to greater damage due to bruising[25]. Many physical properties of potatoes are directly related to tuber size, while density is inversely proportional to size[24]. Relative density is an important indicator of potato quality[26], reflecting dry matter content and water content. This information can be used when designing potato sorting and packaging equipment to reduce losses during transportation and storage. Water content in potatoes is a critical factor, as Surdilovic et al.[25] observed that potatoes experience less force and greater deformation at higher water contents. Since increased deformation means increased potato damage, having a higher relative density is a desirable trait, allowing harvesters to move faster while applying additional force to potatoes without increasing the level of damage. The specific gravity of potatoes is affected by several factors, including harvest time and potato variety. In a study conducted by Waxman et al.[26], three potato varieties were grown at different harvest times. The results of their study showed that lower specific gravity was associated with early and late harvest. Also, the potato variety affected specific gravity. The results also showed that different potato varieties affected the specific gravity, with Clearwater Russet achieving the highest specific gravity in each of the experiment years.
Soil properties
-
Implementing sound agricultural practices for potato cultivars can significantly improve crop quality. These practices and potato characteristics, such as flesh color, influence the nutritional requirements for optimal potato growth and harvest[27]. Furthermore, the demand for and availability of nutrients in the soil change throughout the growth cycle. Soil type and water content play a vital role in minimizing tuber damage and loss during harvest[28]. Heavy clay soils are a particular challenge for harvesting, as they are more susceptible to compaction, resulting in large clods of soil being extracted with potatoes, causing bruising and damage to tubers. Low water content can also increase the likelihood of bruising and damage to potatoes during harvest[6]. Soil water content can be controlled through irrigation systems[29], ensuring optimal potato growth and reducing the risk of water shortages. However, the environmental impact of this irrigation must be taken into account, which calls for minimizing the environmental impact while enhancing long-term yields[29]. On the other hand, soil compaction affects the force required to dig potatoes. Designs that reduce traction resistance, such as the use of passive blades with cutting discs and soil compactors, can improve harvest efficiency by up to 22%[30]. Soil adhesion to tubers is another key factor; designs that incorporate soil-crushing mechanisms can reduce soil adhesion to potatoes by more than 50%[31].
-
Improving the performance of potato harvesters involves adjusting several variable parameters, which are affected by the characteristics of the potatoes and the soil. These parameters include the forward speed and conveyor speed, as well as the digging depth and angle. The forward speed of the harvester ranges from 0.9 to 7.9 km/h, while the conveyor speed ranges from 0.2 to 2.37 m/s. The digging angle ranges from 10° to 24°, while the digging depth ranges from 12 to 27 cm. These parameters play a crucial role in reducing tuber damage during harvesting, with studies showing that the optimum digging depth is 20 cm, and the penetration angle ranges from 18° to 21° to achieve the best results[30].
Soil and potato characteristics are key factors in determining the optimum parameters for potato harvesters, as the farmer must minimize tuber damage and increase harvest efficiency. Research has shown that increasing the forward speed of the harvester significantly affects tuber damage. Bulgakov et al.[28] showed that tuber damage decreased from 4.2% to 1.5% when the forward speed increased from 2.9 to 7.9 km/h. However, other studies have shown contradictory results, with Hrushetsky et al.[32] finding that increasing the speed may increase tuber damage. The forward speed also affects the efficiency of soil-tuber separation. Bulgakov et al.[33] reported that the separation efficiency improved with increasing speed up to a certain point, after which the increase led to a decrease in performance. They observed that the separation efficiency increased slowly up to 3.0 km/h, then decreased at 4.0 km/h. Bulgakov et al.[28] confirmed that increasing the speed from 5.4 to 7.9 km/h resulted in a decrease in soil separation. Finally, Issa et al.[34] found that increasing the forward speed generally increased the actual field capacity but reduced the harvest efficiency and specific energy consumption. While the tuber lifting ratio increased when the speed increased from 2.5 to 4.5 km/h, this ratio decreased when the speed increased from 4.5 to 6.5 km/h.
The relationship between digging angle and depth relates to the effect of angle on digging efficiency and crop quality. Studies show that increasing the digging angle usually results in increasing depth, which can reduce tuber losses by adjusting the digging angle. According to Issa et al.[34], the percentage of lifted potatoes increased from 87.63% to 95.14% when the digging angle increased from 12° to 22°, with a decrease in total damage to potatoes. However, it should be noted that increasing the digging angle also increases soil resistance, which negatively affects the actual field capacity and harvesting efficiency. It also increases the energy required and specific force required to operate the harvester. Therefore, the balance between digging angle and depth is vital to achieve the highest harvesting efficiency and minimize damage caused by digging.
Increasing the conveyor speed negatively affects the damage of potato tubers during the separation process from the soil. Wei et al.[6] showed that different separation stages experienced different levels of soil loosening of tubers. The results showed that changing the potato-to-soil ratio had a significant effect on harvest quality, with the number of bruises increasing with the increase in potato ratio and the decrease in soil cushion. The movement of potatoes on the conveyor varies by stage; in the initial stage, the movement is simple, while in the middle stage, the potatoes roll, and in the final stage, they jump and roll, which increases the possibility of damage. As the vibration intensity increases, the number and acceleration of shocks increase, which requires choosing the appropriate vibration intensity to reduce bruises and mechanical damage while maximizing the efficiency of the separation process.
Studies have shown that increasing conveyor speed has a negative effect on soil separation ratio and separation density, with these ratios decreasing with increasing speed[33]. On the other hand, actual field capacity and harvest efficiency improve with increasing conveyor speed, although changing speed did not have a significant effect on tuber damage. The researchers also found that increasing conveyor speed results in a decrease in the tuber lifting ratio. These results highlight the importance of controlling conveyor speed to balance efficiency and harvest quality[34].
-
Potato harvester design is an area of growing interest, with designs ranging from simple to complex. Simple designs focus on digging depth and speed, while complex designs focus on motors and rotating components to remove soil clods. Designs that maximize efficiency while minimizing damage are most desirable. Among the popular options, the harvester's operational subcomponents play an important role in breaking up the soil and minimizing tuber damage, resulting in increased efficiency and reduced damage. Table 1 shows some recent developments in potato harvester design.
Table 1. Specifications of some types of advanced potato harvesters.
Specifications Advanced harvester Ref. A new potato digger was designed with a negative blade with cutting discs and soil compactors on both sides of the digger, reducing the traction resistance by 18%. This improvement increased the hollowing rate of the potato layer and soil, contributing to a 22% increase in productivity. The new design achieved a yield of 13.2 t/ha and a digging completion rate of 99.1%. In comparison, the KST-1.4 series digger achieved a completion rate of 97.6%, confirming the effectiveness of the proposed design in improving the overall harvesting performance. [32] The potato harvester is designed to harvest two rows of potatoes at once. The working mechanism is based on breaking the soil to expose the potatoes, which are then lifted using a separator conveyor system that includes a chain bar and a hoist conveyor and is operated by a 40-hp tractor. The initial design proved effective, with a field capacity of 0.26 ha/h, a bruising rate of 6%, and a transfer rate of 98.4% of the dug potatoes to the cart with a field loss of 1.6%. [7] A rotary component was added to the design of the potato harvester to improve the soil fragmentation process and reduce the soil masses lifted during separation. The researchers modified several parameters such as the machine's travel speed, rotor rotation frequency, rotor diameter, and the distance between the spherical discs to study their effect on the performance of the device. The results showed a significant improvement in soil separation as the rotor diameter increased from 0.65 to 1.0 m, and as the travel speed increased from 0.8 to 1.5 m/s. [28] The potato pile separator with self-cleaning cylinders was used to improve the soil separation process. The researchers determined the optimum parameters of the spiral separator, which include a peripheral rotation speed of 1.75 to 2.0 m/s, a separator inclination angle relative to the horizon of 15° to 19°, and a screw fixation concentricity of 5 to 10 mm. The researchers also recommended a forward speed of 2.16 to 2.88 km/h. The results showed that increasing the inclination angle and screw concentricity improved the soil screening and separation density. On the other hand, increasing the peripheral rotation speed to about 2 m/s led to a gradual decrease in the sieved soil ratio, where a rapid decrease in this ratio was observed after exceeding 2 m/s. [33] A V-shaped pile distributor has been added to the potato digger to improve the separation process. By distributing the excavated pile evenly across the width of the elevator grid, the separation quality is improved. This has been shown to increase the purity of tubers in the bin to 97.4%, as well as increasing productivity (by 0.16 ha/h). The machine operates efficiently at speeds above 3.55 km/h, exhibiting less tractive resistance than conventional models. [35] A left and right rotation formula was developed for the potato soil separator, with the rotation speed of the separating cylinder being 100 rpm and the center distance of the separating cylinder being 79 mm. The results showed that the bruising rate was 1.25%, the clean potato ratio was 99.01%, and the peel breakage rate was 1.58%. When the inclination angle of the device was set at 8°, the rotation speed of the separating cylinder was reduced to 80 rpm while keeping the same center distance of the cylinder, resulting in an increase in the bruising rate to 1.43%, the clean potato ratio to 98.64%, and the peel breakage rate to 1.77%. [36] As mechanical harvesting techniques for potato crops continue to develop, the problem of impurities such as lumps and stones that resemble potatoes in size and quality remains, complicating the process of separating these impurities from potatoes after mechanical harvesting[37]. This problem is particularly severe in areas with heavy clay soil, where soil viscosity is high, resulting in a high rate of impurities in potatoes after harvest[38]. As a result, more manual sorting and cleaning operations are required, which increases production costs[39].
-
In recent years, domestic and international researchers, as well as agricultural machinery manufacturing enterprises, have conducted a large amount of research on potato cleaning methods and equipment. However, these methods and technologies have not been integrated with potato harvesters. Artificial intelligence is increasingly being applied to post-harvest processing of potatoes, especially in the detection and sorting of tubers, to enhance quality control and reduce food loss. The integration of artificial intelligence technologies, especially machine vision, has revolutionized traditional sorting methods, allowing for more efficient and accurate classification of potatoes based on various criteria. Computer vision systems have been used in agricultural operations for many years to control product quality[40]. These systems rely on high-speed cameras and advanced image processing algorithms to assess the size, shape, and external quality of potatoes. For example, a high-speed potato sorting machine can process up to 12 tons of potatoes per hour, using a 360-degree imaging system to identify defects such as greening, mechanical damage, and diseases such as Rhizoctonia and common scab. The accuracy of these systems is enhanced using techniques such as linear discriminant analysis and Mahalanobis distance classifiers, achieving up to 89.2% accuracy in detecting defects[4].
Sorting machine manufacturers are competing to offer innovative solutions to ensure sorting quality. In this context, an automated control station equipped with a CCD camera was developed to automatically classify potatoes by size and shape. Potato size estimates were based on calculating the Euclidean distance between vertex points using a boundary tracking algorithm, resulting in a diameter prediction error of less than 1.5%. Potato shape was analyzed using Fourier descriptors, with the system's classification accuracy reaching 97%−98% in static tests, but dropping to 77%−88% in dynamic tests, with a classification efficiency of up to 180 t/h[41]. Therefore, there is an urgent need to develop more robust algorithms to improve the accuracy of dynamic classification of moving potato shapes.
A high-speed potato sorting machine was developed to monitor quality for size, shape, and external defects such as greening, mechanical damage, and pathogens. The system uses a three-line CCD camera with an array of mirrors to provide a 360° view of the tuber as it passes through, allowing the images to be processed and graded. The machine operates at a belt speed of up to 1.5 m/s, enabling a processing rate of up to 12 t/h, or about 50 potatoes per second. External defects and diseases were identified by color segmentation analysis using linear discriminant analysis (LDA) and a Mahalanobis distance classifier, with features such as area, eccentricity, and central moment. To detect deformities, a Fourier-based shape transform was used, achieving a shape prediction accuracy of up to 89.2%[42].
A machine vision prototype was developed for real-time sorting and detection of quality parameters of agricultural products (Fig. 3). The system is based on image capture and processing using color value data to detect defects. A simple thresholding method was used, which allowed the detection of various defects in potatoes. The results showed that the accuracy of defect detection was high, reaching 98.50%, reflecting the effectiveness of the model in improving sorting processes and reducing costs associated with manual sorting[43]. Table 2 shows the application of other machine vision systems in light field methods for potato quality assessment.
Figure 3.
Machine vision model developed for potato quality inspection[43].
Table 2. Applications of machine vision systems for potato quality inspection.
Application Methodology Results Ref. Quality inspection High-speed machine vision system for classification based on weight, sectional diameter, shape, and color, using CCD cameras and image processing algorithms. Achieved overall success rates of 86.5% for weight, diameter, shape, and color inspections. [44] Surface defect detection A light chamber and CCD cameras were used to capture images to detect defects such as vegetation and scab. The detection accuracy ranged from 86.8% to 98.6% for various defects. [45] Depth imaging for defect detection Implemented a depth imaging system combined with convolutional neural networks to assess surface defects. Achieved 94.5% accuracy in size classification and 91.6% in appearance classification. [46] Ultraviolet imaging for
tubers detectionDeveloped a machine vision system using ultraviolet imaging to distinguish potato tubers from clods on a conveyor. Enhanced detection capabilities for tuber identification. [47] 3D model rebuilding for feature prediction Employed machine vision with multispectral and hyperspectral cameras for predicting potato features like length and mass. Enabled accurate predictions of physical characteristics, aiding in quality grading. [48] Artificial intelligence technologies, especially non-contact machine vision, are powerful tools for providing accurate information on the mechanical, physical, and chemical parameters of potato tubers. Accurate post-harvest grading of potatoes based on internal defects is vital for storage operations, as early disease detection helps reduce the spread of pathogens, thereby reducing storage losses. For potatoes destined for planting, accurate grading helps reduce the incidence of diseases on farms, enhancing crop quality and yield. With the increasing demand for high-quality products due to market globalization and ensuring food safety, the need for accurate sorting machines to grade agricultural products is increasing[4].
The use of AI is expected to continue to improve post-harvest potato sorting processes, enhancing the quality of the final product. Innovations in machine design will also help increase efficiency, helping farmers achieve higher yields and reduce waste. In this context, it is important to invest in technology to improve production efficiency, as well as to consider local potato and soil characteristics when designing machines. Enhancing collaboration between farmers and manufacturers is vital to developing innovative solutions that meet market needs. Together, these factors contribute to improving agricultural productivity and reducing waste, which enhances food security and brings tangible economic benefits to farmers.
-
There are key differences between manual and mechanical potato harvesting, with mechanical harvesting emerging as a more efficient option, resulting in reduced waste and increased productivity. However, manual harvesting remains a suitable option in certain circumstances, especially in areas where greater precision is required or where machinery is not suitable. The challenges facing mechanical harvesting relate to the design of the machinery, which must consider the characteristics of the potato and the soil. These factors directly affect the overall performance of the machine and its ability to reduce damage. Recent innovations in harvesting machinery have also contributed to improving efficiency and increasing productivity, such as the use of advanced technologies in machine design. In addition, artificial intelligence is playing an increasingly important role in improving post-harvest potato sorting processes, which enhances the quality of the final product. These technological developments point to a promising future in agriculture, as they can contribute to achieving higher productivity while reducing costs. Therefore, choosing the appropriate harvesting method requires a careful assessment of the agricultural and economic conditions. Farmers must consider various factors, such as soil type, crop condition, and costs, to ensure the best results. Ultimately, both manual and mechanical harvesting have advantages and disadvantages, choosing between them depends on the specific agricultural context.
-
The authors confirm contribution to the paper as follows: study conception and design: Al-sammarraie MAJ, Gokalp Z, Ilbas AI; data collection: Al-Sammarraie MAJ; analysis and interpretation of results: Al-Sammarraie MAJ; draft manuscript preparation: Al-Sammarraie MAJ, Gokalp Z, Ilbas AI. All authors reviewed the results and approved the final version of the manuscript.
-
Data sharing not applicable to this article as no datasets were generated or analyzed during the current study.
-
This is a collaborative review, and funding was not available. We would like to thank the University of Baghdad and the Erciyes University.
-
The authors declare that they have no conflict of interest.
- Copyright: © 2025 by the author(s). Published by Maximum Academic Press, Fayetteville, GA. This article is an open access article distributed under Creative Commons Attribution License (CC BY 4.0), visit https://creativecommons.org/licenses/by/4.0/.
-
About this article
Cite this article
Al-Sammarraie MAJ, Gokalp Z, Ilbas AI. 2025. Challenges and innovations in potato harvester design: the role of artificial intelligence in improving crop sorting. Technology in Agronomy 5: e004 doi: 10.48130/tia-0025-0001
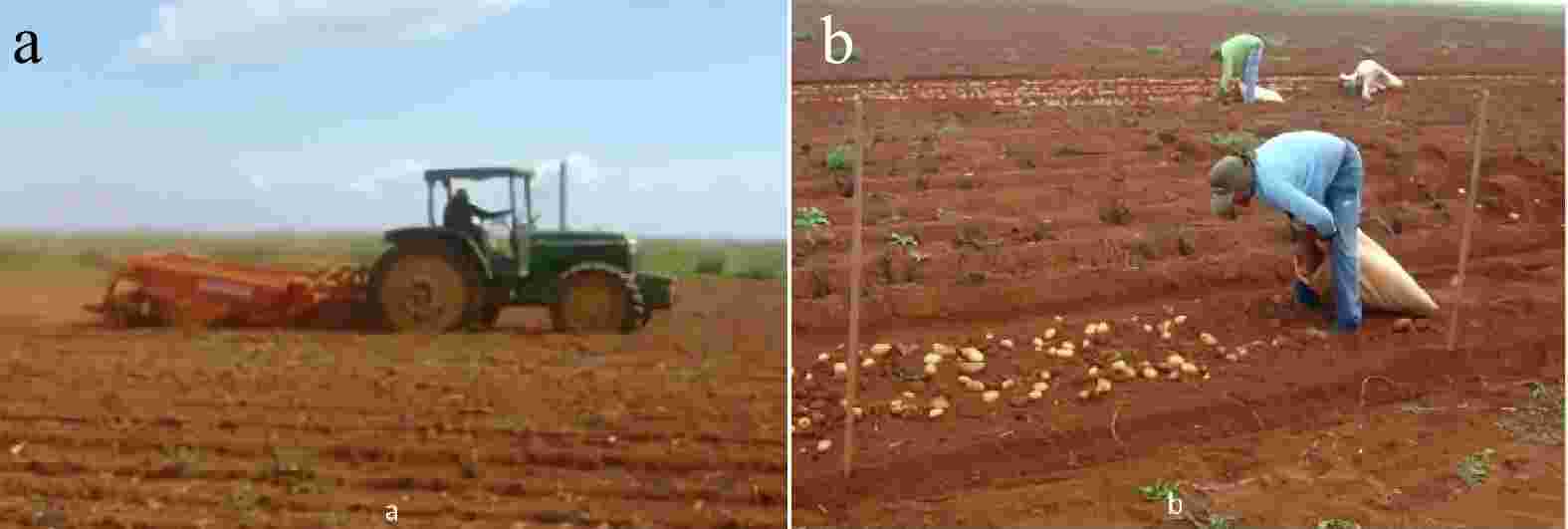