-
The domestication and breeding of crops initiated over ten thousand years ago[1]. The process saw its major transformation with the advent of Mendelian genetics and quantitative genetics. This shift accelerated breeding from simple phenotypic selection (Breeding v1.0) to more sophisticated hybridization (Breeding v2.0). The advent of modern biotechnological tools, such as molecular markers, whole-genome prediction, and transgenic technologies, has ushered in the third generation of breeding. Forward genetics continues to be a pivotal approach for elucidating the genetic basis of critical traits and pinpointing key genetic loci in horticultural crops. Meanwhile, the integration of multi-omics resources is serving as effective accelerators to further promote horticultural crop breeding towards stage 4.0[2].
In plant breeding, the dissection of gene-trait associations has been the foundation for efficient breeding. Forward genetics combines traits with variants using statistical methods to identify key loci associated with specific traits, also known as quantitative trait loci (QTL). Current methods include linkage mapping and association mapping. Linkage mapping relies on recombination events and polymorphic markers generated in experimental populations to identify QTLs based on genetic linkage maps. Genome-wide association studies (GWAS) leverages the principle of linkage disequilibrium, using historical recombination accumulated across panels of essentially unrelated individuals to assess the correlation between phenotypes and genetic markers.
With the reduction in sequencing costs and a significant increase in throughput, omics data are now extensively applied in plant research. Multi-omics studies offer a comprehensive analysis of biological processes, encompassing genomics, transcriptomics, proteomics, metabolomics, and epigenomics[3]. Increasingly, research integrates multi-omics techniques with traditional forward genetics, increasing efficiency and precision in variant detection, genetic mapping, gene discovery, and regulatory network construction[4]. At the same time, artificial intelligence (AI) and machine learning (ML) also provide powerful tools for multi-omics data processing, enabling predictive models in genome selection and breeding[5], high-throughput phenotyping[6], etc. Such strategies add new vitality to modern plant biology, facilitating our understanding of biological phenomena and mechanisms.
This review summarizes studies that use multi-omics data to assist mapping for important traits in horticultural crops, while proposing how such methods can be applied in ornamental plants, along with their precautions. We wish to provide concrete examples of how to efficiently combine multi-omics data with forward genetics, aiming to explore the relationship between genes and traits in different dimensions.
-
Since the publication of the first Arabidopsis thaliana genome in 2000, plant genomics have rapidly developed over the past two decades[7]. The publication of the grapevine (Vitis vinifera) genome in 2007 marked the beginning of the genomic era for horticultural crops[8]. Plum blossom, also known as mei (Prunus mume), was the first ornamental plant to have its reference genome assembled[9]. With advancements in sequencing and assembly technologies, plant genomics has shifted its focus from establishing reference genomes to unveiling complex biological questions related to plant origins, evolution, domestication, and trait dissection through comparative genomics[10].
Reference genomes are essential for detecting variations
-
In population genetic analyses, short-read sequences have to be accurately aligned to a reference genome for variant calling and genotyping. A genome-wide variation map not only characterizes genetic diversity, it also serves as a foundation for the analysis of key agronomic traits. Thus, the quality of the reference genome significantly influences mapping results. High-quality reference genome assemblies, combined with linkage mapping and association analysis, have efficiently aided in dissecting key traits in fruits[11−14], vegetables[15], ornamental and medicinal plants[16,17]. In the association study of peach fruit traits, Tan et al.[12] assembled five Prunus reference genomes for variant calling of 417 accessions. They further discovered an inversion of the OVATE transcription factor controlling fruit shape and duplications of the NAC transcription factor controlling fruit development.
Using genome annotation to locate candidate genes
-
The process of genetic mapping can be both laborious and time-consuming. Nevertheless, as genome information becomes more accessible, studies have efficiently identified candidate genes within QTL intervals using genome annotations. Wei et al.[18] used the annotation information of a high-quality eggplant (Solanum melongena) genome to identify a candidate gene, Smechr0301963, within a ~7 Mb (71.29−78.26 Mb) interval. A GWAS analysis on six fruit quality traits in longan (Dimocarpus longan) cultivars resulted in a single co-localized QTL interval[19]. Three candidate genes associated with total soluble solids and seed weight were revealed using the genome annotation of longan 'JDB' reference genome. Zhou et al.[20] used both genetic mapping and bulked segregant analysis (BSA) results to identify the gibberellin-related GID1b gene related to plant height, assisted by the annotations from the high-quality Lagerstroemia indica genome.
T2T gap-less genomes assist fine-mapping
-
Gaps and errors during genome assemblies are inevitable in most cases, which can result in inaccurate variant identification and consequently interfere with downstream analyses[10]. Nowadays, the long-read sequencing technologies have enabled the near-complete assembly of each chromosome (i.e. telomere-to-telomere assemblies)[21], which has been applied in several horticultural crops[22−26]. This achievement allows for more refined and accurate detection of genetic variants across the entire genome and population. Using the watermelon (Citrullus lanatus) line G42, Deng et al.[23] assembled a T2T genome and detected a nonsynonymous mutation ClFS1 related to fruit shape. Fu et al.[22] identified structural variants (SVs) and presence-absence variants (PAVs) related to fruit quality, terpene metabolism, and trait evolution based on the T2T genome of bitter melon (Momordica charantia var. abbreviata). Li et al.[27] conducted variant calling and GWAS analysis utilizing the T2T reference genome of melon (Cucumis melo). Using different melon lines, they identified a significant SNP related to fruit ripening and successfully mapped a candidate gene CmABA2.
Reference genome assemblies of ornamental plants
-
We summarized studies on the genome assemblies of ornamental plants from 2012 to the present (Supplemental Table S1). The main objectives of ornamental plant genome research include the dissection of characteristic metabolic pathways (such as floral fragrance, flower color, hormones, etc.), as well as the exploration of genes involved in floral development, flowering time regulation, etc. Before 2017, most genomes were based on short-read sequencing for genome assemblies, resulting in many genomes remaining at the draft level. Since 2018, with the popularization of third-generation sequencing technology, the quality of ornamental plant genomes has generally improved. In recent years, a total of seven ornamental plants have achieved T2T-level genome assembly[28−34]. These studies provided insights into mechanisms of flower color, flower scent, and disease resistance. However, due to repeated hybridization and genome duplication events, many ornamental plant genomes are large and highly heterozygous, leading to challenges in genomic research for these species. At present, most ornamental plant genomes are still merged genomes (i.e. not phased or haplotype-resolved) with a lack of information regarding allelic variants. In addition, we found that the plant materials used for genome assembly are mostly wild and diploid species, which may lack representative traits unique to certain varieties. Among all these materials, polyploid species only accounted for 8.5%. A recent study has made significant progress in assembling the hexaploid chrysanthemums genome[35], delving into the origin and breeding history of cultivated chrysanthemums, and laying the foundation for further studies of important ornamental traits (flower shape, color, plant architecture, stress resistance, etc.). Therefore, future studies for ornamental plant genomic research may focus more on representative varieties with target ornamental traits. In addition, it is necessary to further overcome the phasing difficulties of polyploid genomes to reveal allelic variations with haplotype-specific genome assemblies.
Genome re-sequencing: genomic variations for all
-
Variant calling is a fundamental step in mapping genetic traits within populations. As more species have completed reference genome assemblies, whole genome re-sequencing (WGS) is becoming increasingly popular in both breeding populations and natural populations. The rapid development of WGS technology has constructed genomic landscapes for various species, which makes variants more accurate and comprehensive. These variants can be valuable in the evaluation of genetic diversity, and population genomics, and can be applied in marker-assisted breeding.
Re-sequencing in breeding populations
-
In breeding populations of horticultural crops, genome re-sequencing has improved the marker density and coverage of genetic linkage maps, continuously refining QTL mapping results[36−38]. For example, the density of grape genetic maps has been increasing, which has led to rapid progress in mapping important traits such as berry shape, berry color, and aroma[37,39,40]. Based on simplified genome sequencing and genetic map construction, a PyPPCD1 gene related to pear (Pyrus spp.) russet fruit skin was fine-mapped and identified[41]. Similarly, a WGS-based high-density genetic map of pear mapped multiple QTLs for fruit quality traits[38]. In experimental populations of ornamental plants, such as modern roses (Rosa spp.) and ornamental kales (Brassica oleracea var. acephala), the marker density of the genetic linkage maps has been greatly increased by WGS technology, laying the foundation for further fine-mapping studies of important ornamental traits[42,43].
Re-sequencing in natural populations
-
WGS technology is also driving advancements in population genetics of natural populations. Song et al.[44] provided a detailed summary of the research progress on plant population genome re-sequencing, as well as its applications and prospects in population genetics. The evaluation of genetic diversity, population structure, and reconstruction of dynamic history based on genome-wide variations can provide a basis to study crop evolution and improvement. Many important horticultural crops have utilized WGS based on extensive material collections to trace the domestication history and identify key domestication genes. In a recent study, Dong et al.[45] reconstructed the historical events of grape origin and domestication process based on genome-wide information from 3,525 grape genetic resources worldwide. Taking roses as a representative example, studies have revealed that genetic diversity and breeding history of roses using a wide range of accessions, meanwhile identifying selection loci related to domestications[46,47]. In addition, WGS and SNP calling in specific hybrid populations or wild accessions can be applied in developing high-density SNP arrays, which can be widely used in marker-assisted breeding in horticultural crops. Wei et al.[48] developed the 200K SNP array for tea (Camellia sinensis), using the 'Longjing 43' genome as a reference and 139 re-sequencing accessions of Camellia resources. Based on this SNP array, the authors constructed a high density genetic linkage map of tea plants and two major loci controlling leaf size were located. Meanwhile, genotyping based on SNP arrays has advantages such as low cost, accuracy, and efficiency, especially for polyploid species. Various polyploid horticultural crops have high-density SNP arrays for marker genotyping, such as kiwifruit (Actinidia spp.)[49], roses (Rosa spp.)[50], potato (Solanum tuberosum)[51], and sugarcane (Saccharum spp.)[52], etc.
Re-sequencing in ornamental plant populations
-
The studies of population genetics in ornamental plants based on whole genome re-sequencing were summarized (Supplemental Table S2). It was found that the majority of these studies have been conducted within natural populations, rather than breeding populations, which may be due to the heterozygous and polyploid backgrounds of most ornamental plants, making it difficult to construct artificial populations. Notably, significant achievements have been made in mapping ornamental traits in numerous important woody plant species, including Prunus mume, Rosa spp., Osmanthus fragrans, Prunus persica and Prunus campanulata, through the utilization of extensive germplasm collections and population genetic approaches. However, in comparison to horticultural crops, several considerations should be considered for future studies in ornamental plant population genetics:
i) Population size. According to Hardy-Weinberg principles, the size of a population significantly influences linkage disequilibrium (LD) decay, which in turn affects the accuracy of mapping results. The present review indicates that only 9 out of 23 studies included populations exceeding 200 individuals (Supplemental Table S2). Therefore, population studies in ornamental plants must encompass a more extensive and comprehensive collection of plant materials to ensure robust and precise mapping results.
ii) Identification of plant materials. The classification of species, variants, and varieties is complex in ornamental plants, which can easily lead to inaccurate identification of plant materials and resulting in unreliable results.
iii) Grouping of plant materials. Compared to horticultural crops, ornamental plants have experienced large hybridization events in a short period of time, resulting in unclear differentiation between wild species, landraces, and varieties. Consequently, it is necessary to consider how to scientifically group these materials (based on sampling regions/genetic relationships/breeding events, etc.), further evaluate the degree of differentiation between different groups, and then conduct subsequent analysis.
Pan-genome as the new reference
-
Plant genomes exhibit both conservation and diversity. Although the assembly of a single reference genome for the species can characterize genetic diversity at a given scale (e.g., SNPs, small InDels), it represents a limited range of genetic variation. Increasing evidence shows that large structural variations (SVs) play a crucial role in plant adaptive evolution and functional diversity[53]. Genome-wide SVs show powerful potential in GWAS analysis, possibly leading to the discovery of more novel loci related to important traits[54]. Plant pan-genomes can capture the genetic diversity of almost the entire spectrum of certain species and their wild relatives, therefore more comprehensively reflect genetic information, and present a complete picture of population genomics. Despite the nascent stage of pan-genome research in horticultural crops, which is subject to a multitude of factors including genome complexity, analytical methodologies, software applications, and genotyping approaches, significant progress has been made in various horticultural crop species, including pepper (Capsicum spp.)[55], tomato (Solanum lycopersicum)[53,56,57], citrus (Citrus spp.)[58,59], rapeseed (Brassica napus)[60], apple (Malus spp.)[61] and sunflower (Helianthus annuus)[62]. We have compiled an overview of the current pan-genome research conducted in horticultural crops, as detailed in Supplemental Table S3. The predominant assembly strategies employed in these studies encompass 'map to pan', de novo assembly, and graph-based pan-genome approaches.
Detecting large-scale variations
-
Utilizing a pan-genome as reference can reduce misalignment caused by linear reference genome and reveal large-scale variations that may associate with agronomic traits. Zhou et al.[57] used both single linear genome and pan-genome as references to detect SVs in tomatoes. It is proved that SVs are important sources of heritability and the ability of a pan-genome can increase the identification of heritability by 24%. Li et al.[53] constructed a tomato graph-pan-genome by assembling the genomes of 11 chromosome-scale wild and cultivated tomato species. These genomes covered the major branches of the tomato's wild relatives and their corresponding cultivated varieties. By integrating previously reported tomato genomes and SV variations, they genotyped SVs in a tomato population with 321 accessions. Furthermore, an SV-based GWAS analyses on 32 flavor-related compounds and 362 fruit metabolic products was conducted, detecting significant association signals for 17 flavor volatiles and 249 fruit metabolic products. Polyploid plants face greater challenges in pan-genome assembly. Song et al.[60] assembled and annotated eight representative allotetraploid rapeseed genomes and created a comprehensive variation map of the rapeseed pan-genome, including SNPs, InDels, PAVs, and homologous exchanges (HE). They employed a PAV-based genome-wide association study (PAV-GWAS) for three yield-related traits and identified PAVs that cause functional gene variations. The study also proposed a strategy of combining SNP-GWAS and PAV-GWAS to detect loci associated with target traits at different variation levels, offering a novel methodology for association analysis of important crops.
Tracing origins and domestication history
-
The assembly of a pan-genome using materials with different genetic backgrounds can effectively trace key genes related to crop domestication and breeding events. A graph-based pan-genome for the orange subfamily (Aurantioideae) and the genome sequences of 314 accessions revealed the origin center of the Citrus genus[58]. By compiling gene expression results, researchers identified a CitPH4 gene, involved in citric acid accumulation during Citrus domestication. In a recent study of pepper, three accessions were selected to represent three distinct clades of cultivated Capsicum, for sequencing and assembly of pepper reference genomes[55]. Based on a graph-pan genome and re-sequencing of 500 Capsicum accessions, differentiated genomic regions and domestication sweeps were identified for fruit size, shape and pungency traits.
Pan-genomes of ornamental plants
-
Currently, among ornamental plants, mei and sunflower have taken the lead in completing pan-genome constructions[17,62]. Targeting important ornamental traits and resistant traits, studies revealed several important loci at the population scale. The materials used in both studies were relatively comprehensive, covering wild species, closely related relatives, landraces, varieties, and mapping populations. However, limited by the sequencing technology, their reference genomes and pan-genome assemblies were based on Illumina short-read sequencing. Thus, the quality of the reference genomes was not guaranteed and they were not capable of identifying large-scale variations. At present, many ornamental plants, such as roses and chrysanthemums, are expected to further promote pan-genomic research with many genomic resources gradually published. At the same time, taking close wild relatives into pan-genome analyses can provide additional genetic diversity, which is the key to breaking through the genetic bottleneck of ornamental plants in future breeding works. In addition, it should be aware that polyploid genomes may have a larger proportion of variable genes and core genes, together with high levels of tandem repeats (TE), which may affect the accuracy of detecting large variants. Thus, it is still challenging to effectively cover the information of polyploid genomes during the pan-genome construction.
-
Traditionally, QTL refers to the quantitative trait loci associated with phenotypic traits. Nowadays, the concept of 'trait' has been broadened to encompass multi-faceted biological data, such as gene expression profiles, protein abundance levels, and differential metabolite concentrations (Fig. 1). Numerous studies have demonstrated the utility of integrating these diverse datasets through linkage or association analyses, to elucidate plant biological mechanisms at different levels.
Mapping expression data
-
Expression quantitative trait loci (eQTL) are genomic loci that control the expression levels of quantitative traits. Genome variations such as SNPs, do not necessarily affect the expression abundance of certain mRNAs or proteins. Only genes that are expressed and translated may have a direct influence on phenotypes. This has led to the exploration of mapping another dimension of data, expression data of mRNA. eQTL analysis associates genetic variation with transcriptomic datasets, which can be served as a powerful tool to understand gene expression variations between individuals[63]. With recent development, eQTL analysis has been used for association analysis and construction of regulatory networks in various horticultural crops, such as tomato[64], strawberries (Fragaria × ananassa)[13], peony (Paeonia suffruticosa)[65], sweet potato (Ipomoea batatas)[66].
In rapeseed, multiple studies have combined transcriptome data to conduct association analysis and locate seed oil content (SOC) traits. Tang et al.[67] performed both GWAS and transcriptome-wide association study (TWAS) to construct a gene regulatory network related to SOC using rapeseed inbred lines. Tan et al.[68] combined eQTL analysis with transposase-accessible chromatin sequencing (ATAC-seq) to predict the mechanisms affecting the SOC regulated by eQTL hotspot and identified two transcription factors positively regulate the SOC in rapeseed. Zhang et al.[69] integrated GWAS and TWAS methods to systematically analyze the genetic basis of seed coat percentage in 382 rapeseed accessions.
In octoploid strawberries, researchers integrated multi-omics to detect SNPs and SVs that may affect strawberry flavors[13]. Combining GWAS and eQTL analysis, they focused on the co-localized intervals and further identified key genes related to flavor traits. This integrative approach effectively combined various genetic and genomic tools to elucidate the complex genetic architecture underlying strawberry flavors.
On the other hand, eQTL approach has been relatively limited in ornamental plants. Compared to crops like rice and maize, the construction of mapping populations required for eQTL analysis is still challenging for most ornamental plants, due to their complex and polyploid genetic backgrounds. In addition, the largely clonal propagation of ornamental crops leads to a lack of natural genetic recombination, limiting the genetic variation for eQTL analysis. Meanwhile, the high cost of transcriptome sequencing and data analysis can also be a significant barrier. In a recent study of peony, Peng et al.[65] utilized 24 phenotypic traits and transcriptome data for 119 varieties. They conducted a population association analysis and identified a total of 86 GWAS-related cis-eQTLs and 3,188 trans-eQTLs. These eQTLs were found to be associated with traits such as the number of petals, stamens, and carpels in peonies. However, the results of eQTLs are easily lost in the vast amount of data. Therefore, it is more accessible to perform such analysis on a single gene with clear functions, to identify regulatory loci and construct expression networks for specific pathways.
Mapping metabolites
-
Metabolites are more stable and exhibit higher heritability and consistent diversity in plants, making them ideal traits for population genetics studies. Mapping metabolite quantitative trait loci (mQTL) has been widely used over the past 15 years to dissect the genetic architecture of various metabolites in plants. There have been numerous studies on integrated metabolomics for genetic mapping in horticultural crops, which involved primary and secondary metabolites. These studies mainly focused on flavor, color, and nutrient-related traits.
Flavor traits are important but complex quantitative traits in horticultural crops, encompassing dimensions such as taste and aroma. Numerous studies have explored the impact of specific metabolites on flavors based on targeted/widely targeted metabolomics, and have used genetic mapping or association analysis to locate genes that affect flavor traits in populations, promoting quality improvement of horticultural crops. Szymanski et al.[70] conducted transcriptome and metabolome analysis on 580 lines derived from wild and cultivated tomato varieties. By integrating eQTL and mQTL analysis, it revealed a key pathway during fruit development and ripening, that the α-Tomatine was converted into saponins. Yuan et al.[71] carried out LC-MS/MS analysis to identify a total of 346 metabolites in watermelons (Citrullus lanatus). MGWAS was then performed on these metabolites, and 29 candidate genes were predicted to be involved in regulating fruit taste and resistance quality.
The metabolic traits may also be influenced by human selection. Cao et al.[72] systematically elucidated the changes in the metabolic profiles of peaches during their evolutionary process through comprehensive metabolomic analysis, mGWAS analysis, and eQTL analysis. Meanwhile, the study also speculated that the preference for peach fruit flavors by eastern and western breeders and consumers may lead to different anticancer activity among peach varieties. By integrating population genetic analysis and metabolomic analysis, Lin et al.[73] characterized significant SNPs using mGWAS in 292 apple accessions, revealing fruit quality changes in the apple domestication process.
Ornamental traits such as floral pigments and volatiles are involve in multiple metabolic pathways. Therefore, conducting mQTL analysis in ornamental plants can effectively reveal the genetic patterns of metabolic-related traits. In roses, metabolic analysis has been conducted to reveal the pigment composition in rose petals. Together, GWAS or QTL mapping analysis have been conducted to identify the major QTLs controlling pigment content traits[43,74,75]. It should be noted that due to cost constraints, limited research conducts biological repeats on metabolic measurement within populations, thus the mapping results need to be further validated.
Mapping translation profiles
-
According to the central dogma of molecular biology, transcription of DNA into RNA requires further translation into proteins with specific amino acid sequences to perform functions. The abundance of protein expression is influenced by genotype × environment (G × E) interactions, which can be analyzed through plant proteomics and protein quantitative trait loci (pQTL) mapping[76]. However, the capability of specific plant proteomics studies depends on the quality and availability of genomic annotation information[77], and the analysis of the relative abundance of protein spots can be laborious at the population level.
With the development of biotechnology and proteomics, pQTL analysis has been attempted in crops such as maize (Zea mays) in recent years[78,79], yet the applications of pQTL in horticultural crops are still very limited. In a study on the fruit traits of chili peppers, a high-density genetic linkage map was first constructed based on an RIL population for QTL mapping[80]. Then transcriptome and proteomic data were combined to perform TWAS and proteomic association analysis (PWAS) on fruit color, fruit length, fruit width, and fruit weight. The identified hotspot protein regions were integrated with eQTL regions, and 12 significant QTL loci with related candidate genes were screened for pepper fruit traits.
Although pQTL analysis combined with genomic, transcriptomic, and metabolomic data can effectively explore gene regulatory and translational networks, dissecting the contributions of genetic factors and environmental influences in pQTL studies is still challenging. Moreover, pQTLs may exhibit varying effects in different plant tissues or cell types, adding to the complexity of the analyzing process. Until now, such application remains blank in ornamental plant research. One of the many reasons is that ornamental plants are still in the early stages of genomic research, with incomplete genomic annotation information, which limits the depth of proteomics research. Additionally, proteomics experiments are costly and demand large sample sizes, which also limits their application.
Mapping epigenetic level
-
Epigenetics modifies and regulates gene expression without altering the variation in DNA sequences, which plays a crucial role in plant growth and development. These epigenetic modifications mainly involve DNA methylation, histone modifications, and small RNA (sRNA) mediated modifications[81]. Recent advancements in technologies such as bisulfite sequencing (BS-Seq) and methyl C sequencing (methyl C-Seq) have significantly enhanced our ability to predict DNA methylation status across the entire genome. Furthermore, genome-wide bisulfite sequencing (WGBS) has enabled the construction of high-resolution genome-wide DNA methylation maps at the single-base level. These breakthroughs have not only deepened our understanding of epigenetic mechanisms but also opened new avenues for exploring the intricate relationship between epigenetic and plant biological processes.
Epigenetic quantitative trait loci (epiQTL) refer to the genetic loci that influence epigenetic traits, focusing on the association between epigenetic markers (such as DNA methylation, histone modifications, etc.) and epigenetic traits. Similar to GWAS, epigenome-wide association studies (EWAS) have proven effective in exploring the impact of epigenetic modifications on phenotypes when whole-genome epigenetic markers are available. Comprehensive reviews have summarized studies and challenges in mapping epigenetic loci in plants and their application prospects in crop improvement[82]. Quantitative epigenetics is still advanced in plant research and predominantly conducted in model plants like A.thaliana[83,84], while also making progress in horticultural crops in recent years.
Long et al.[85] first analyzed epiallelic loci in the rapeseed genome, employing methylation-sensitive amplified fragment length polymorphism (MSAP) and retrotransposon (RT) epigenetic markers. They discovered that 90% of the markers exhibited stable allelic patterns across different environments or developmental stages and identified QTLs associated with seven important traits in a mapping population. A recent study in tomatoes has advanced the field of population epigenetics in horticultural crops. Guo et al.[86] conducted WGBS, RNA-seq, and metabolomic analyses in a tomato population. They identified large differentially methylated regions (DMRs) while revealing a strong association of DMRs with adjacent SNPs. Furthermore, the study also performed mGWAS based on both SNPs and DMRs. Integrating multi-omics data, they identified 13 candidate genes and updated the polyphenol biosynthesis pathway, indicating that DNA methylation changes might be a genetic basis for plant metabolic diversity.
In ornamental plants, studies have shown that ornamental traits such as flower color and scent may be regulated by methylation sites. In osmanthus (Osmanthus fragrance), the expression levels of genes related to aromatic components, such as TPS2, CCD4, and CCD1, are associated with methylation levels and chromatin accessibility[87]. In chrysanthemums (Chrysanthemum morifolium) it is proved that the methylation level of CmMYB6 promoter affects anthocyanin accumulation and can be inherited by offspring through mitosis[88]. Such stably inherited loci with phenotypic diversity can be potential targets for natural/artificial selection for ornamental plants improvement.
Mapping high-throughput phenotypic data
-
Plant phenotyping is a comprehensive assessment of complex traits such as development, growth, resistance, yield, etc. Traditional phenotyping methods are costly, labor-intensive, destructive, and may affect the accuracy of mapping results. Over the past decade, ground and aerial platforms equipped with multiple sensors have been rapidly adopted for phenotyping various traits throughout the developmental stages of crop plants[6]. High-throughput phenotyping (HTP) based phenomes are now widely applied for analyzing plant and animal phenotypes at the cellular, organ, individual, and population levels, providing comprehensive and repeatable data[89]. Many crops have integrated HTP with High-throughput genotyping (HTG) and located genomic loci associated with different traits based on linkage and association analyses, advancing genomic selection in crops. Lorence[90] outlined the most commonly used sensors in plant phenotyping studies and summarized plant identification and characterization methods. Yang et al.[91] detailed the development of HTP technologies in crops over the past decades. The technology has great potential in population analysis for complex agronomic traits in horticultural crops.
Wageningen University developed a phenotyping device equipped with RGB cameras and real-time depth sensors, SPICY[92]. The system is capable of capturing plant images with intervals of 5 cm, at 3-m height. Utilizing such a system in a RIL population of peppers comprising 151 genotypes, researchers successfully identified three QTLs associated with leaf size and one related to leaf angle. This technology represents an advancement in phenotypic measurement in horticultural crop populations, offering enhanced precision and depth in trait analysis and QTL mapping.
In rapeseed, the dynamics of growth were captured through HTP technology, yielding phenotypic data on traits relevant to early growth stages, including biomass, early plant height, and mean color values[93]. Combining GWAS analysis, the study identified several major loci associated with biomass and further pinpointed candidate genes involved in meristem development and cell wall modification. The results also showed that early growth of plants is a highly complex trait controlled by several medium effect loci and many minor effect loci. Zhang et al.[94] employed a continuous detection on rapeseed natural populations under different salt stress using HTP platforms and imaging techniques such as hyperspectral and RGB. Rich image-based traits were obtained during given development stages. GWAS analysis based on these traits identified a large number of candidate genes and QTLs related to salt stress, providing a genetic basis for the improvement of salt tolerance in rapeseed.
HTP holds vast potential for dissecting and analyzing many ornamental traits due to its advantages in processing complex traits. By utilizing image-based phenotyping technologies, studies have analyzed traits such as plant architecture[95], flower color[96] and flower intensity[97] in ornamental crops. A noticeable example was using the 3D digitalization method to analyze rose bush architecture[95]. Researchers phenotyped two segregating rose bush populations by 3D digitalization, and then selected the most relevant variables for QTL analysis.
The data landscape generated by HTP omics is high-dimensional and complex, presenting an ongoing challenge in developing corresponding software and algorithms. Appropriate statistical and computational methods are needed for dimensionality reduction, data filtering, and integration, in order to efficiently obtain useful information. Meanwhile, as HTP can elucidate correlations between traits and genotypes, deriving biological patterns and functional studies from these correlations remains challenging. Artificial intelligence and machine learning are now promising powerful solutions for dissecting complex patterns in these large datasets, enabling more accurate plant phenotype models while significantly cut the redundant processing time[98].
Taken together, by employing multi-omics technologies across various genetic resources, researchers can obtain a comprehensive perspective spanning from the genome to the transcriptome, proteome, and metabolome, thereby delving into the intricate networks and interactions of plant biological systems. This integrative approach not only provides a multi-dimensional insight into plant phenotypes, but also enables the capture of dynamic changes in plants under varying developmental stages and environmental conditions, fostering a better understanding of the relationship between environment and genotype. Furthermore, comparing multi-omics data from different populations allows for the identification of molecular markers associated with specific traits. These functional markers are of great significance for the breeding and genetic improvement of horticultural plants, enhancing the precision and efficiency of the breeding process. Through this comprehensive application, we can better shape the systematic understanding of plants, thereby addressing global food demands and improving the yield, disease resistance, and adaptability of horticultural crops.
-
The rapid advancement of next-generation sequencing technology provides a wealth of genetic data. This coupled with the ongoing evolution of omics methodologies has unlocked opportunities to reveal the origins, domestications, and genetic mechanisms of key traits in horticultural crops.
As ornamental plants are increasingly gaining attention, some flowers have been considered as model species for studying crucial traits, leading to the growing application of omics technologies in ornamental plants. However, mapping in populations requires years of material collection and population construction, which is one of the main challenges that limits such studies. Wide germplasm collection and accurate phenotyping are still prerequisites for genetic analyses of complex ornamental traits. Currently, the increased sequencing throughput and the complexity of integrating multi-level omics data present theoretical and computational challenges. Moreover, the genetic transformation system for many ornamental plants requires further optimization to validate mapping results effectively.
Mapping multi-omics data at the population level characterized by its broad scope, high resolution, and efficiency, will undoubtedly accelerate the breeding process of ornamental crops. With new techniques such as single-cell transcriptomics and 3D genomics, more dimensional data will be available to broaden our understanding of genetic regulation in plants. Thus, developing robust algorithms and integrating with AI and ML frameworks for efficient data processing is urgent and necessary. Future interdisciplinary collaboration can realize the transformation from research to new plant varieties, and enhancing the theoretical foundation and practical application of ornamental plant breeding. Together with enhancements in gene editing and genetic transformation systems, it is conceivable that the field of breeding ornamental plants will transition into the 4.0 era of precise and efficient molecular breeding within the near future.
-
The authors confirm contribution to the paper as follows: writing – original draft, reviewing, and editing: Cheng B; visualization and investigation: Du W; data curation and supervision: Bourke PM; conceptualization, supervision and funding acquisition: Yu C. All authors reviewed the results and approved the final version of the manuscript.
-
Data sharing not applicable to this article as no datasets were generated or analyzed during the current study.
This research was supported by the National Natural Science Foundation of China (32071818).
-
The authors declare that they have no conflict of interest.
- Supplemental Table S1 Summary of ornamental plant genome assemblies.
- Supplemental Table S2 Summary of ornamental plant population re-sequencing studies.
- Supplemental Table S3 Summary of pan-genome studies of horticultural crops.
- Copyright: © 2024 by the author(s). Published by Maximum Academic Press, Fayetteville, GA. This article is an open access article distributed under Creative Commons Attribution License (CC BY 4.0), visit https://creativecommons.org/licenses/by/4.0/.
-
About this article
Cite this article
Cheng B, Du W, Bourke PM, Yu C. 2024. Population genetics of horticultural crops aided by multi-omics technology and its implications for ornamental plants. Ornamental Plant Research 4: e024 doi: 10.48130/opr-0024-0020
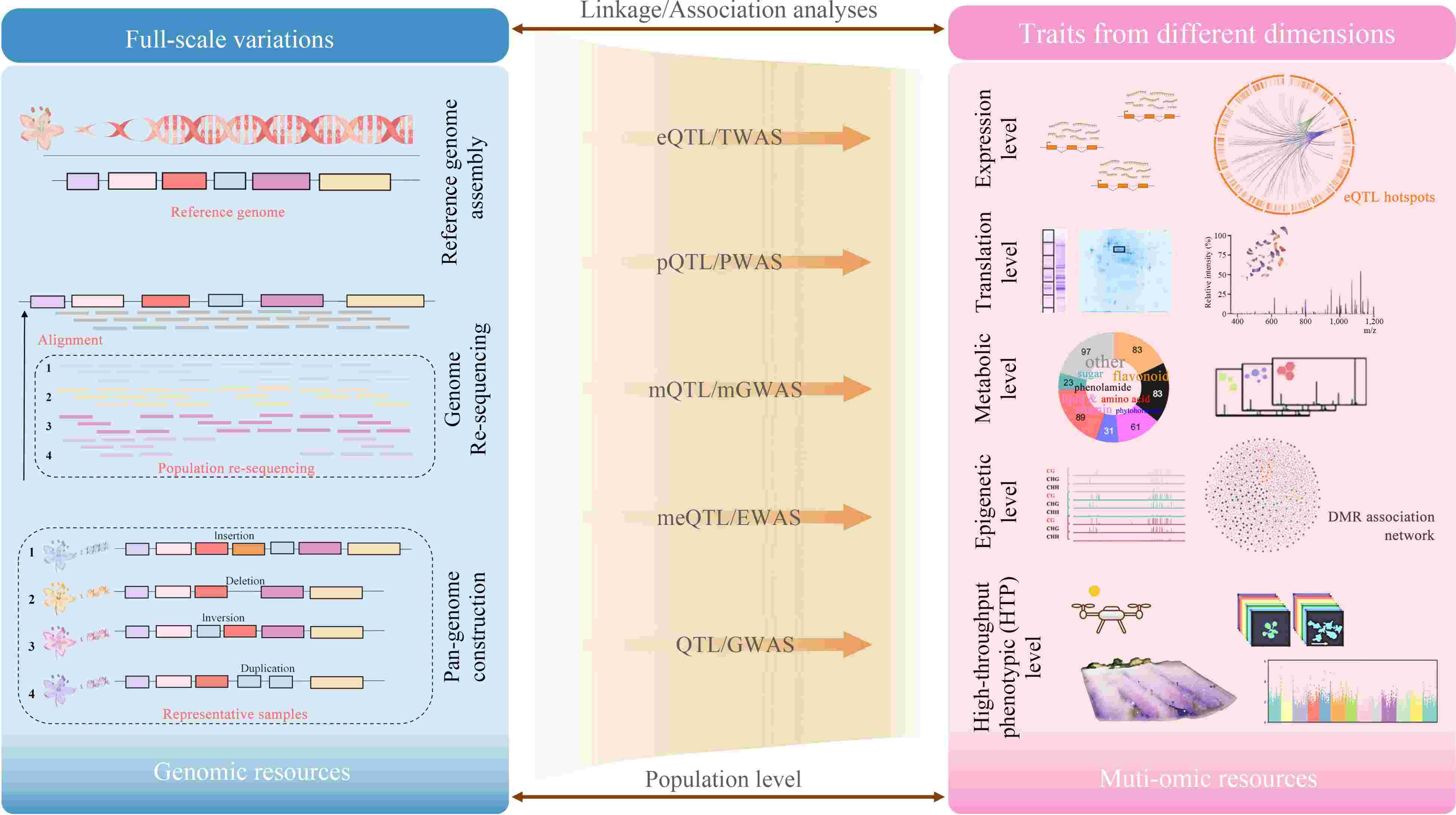