-
Microbial communities play a critical role in plant health and productivity, particularly in enhancing disease resistance, a function that has gained increasing attention in recent years[1]. Root-associated microbial communities promote plant growth through various mechanisms, including the production of antibiotics, antifungal compounds, and secondary metabolites. These substances work synergistically to prevent pathogen invasion in the root zone. For instance, species such as Bacillus and Pseudomonas secrete antifungal and antimicrobial agents in the rhizosphere, strengthening plant defenses against a broad spectrum of pathogens[2,3]. Streptomyces, an important genus within the phylum Actinobacteria that commonly inhabits the rhizosphere, possesses potent antimicrobial and antifungal properties, effectively suppressing a wide range of root-borne pathogens. Furthermore, Streptomyces enhances nutrient uptake, thereby boosting overall plant health and vigor[4]. In addition to disease prevention and promoting plant health, microbial communities enhance overall plant well-being by optimizing nutrient uptake and modulating hormonal balance. Emerging research highlights the critical role of beneficial microbes, such as nitrogen-fixing rhizobia (e.g., Sinorhizobium meliloti) and phosphorus-solubilizing bacteria, in improving soil fertility and optimizing the uptake of essential nutrients like nitrogen and phosphorus[5,6]. Furthermore, certain endophytic bacteria, such as Sphingomonas sp. LK11, promote plant growth by producing phytohormones, including gibberellins and indole-3-acetic acid. These hormones stimulate root and shoot development, leading to significant improvements in key plant traits, such as stem elongation, chlorophyll content, and root and shoot biomass[7]. Microbial communities also play a pivotal role in enhancing plant stress resilience, particularly by increasing plant tolerance to both environmental and biotic stressors[8]. Beneficial microbes significantly bolster plant resistance to soil-borne pathogens, pests, and other environmental pressures. Well-characterized bacterial species, such as Bacillus and Pseudomonas, are known to induce systemic resistance in plants, thereby strengthening their defense mechanisms against a wide range of pathogens[9]. Additionally, microbes like Sphingomonas and Streptomyces contribute to disease suppression through direct antagonism of pathogens or competitive exclusion of harmful microorganisms[10,11]. These beneficial microbial interactions not only enhance plant resilience but also improve crop yields, while simultaneously reducing the need for chemical inputs, thus promoting more sustainable agricultural practices.
While the role of microbial communities in disease resistance is well-documented, their impact on other critical aspects of crop production, such as fruit quality, remains relatively underexplored[1,12]. Fruit quality—encompassing traits such as size, sweetness, texture, and nutritional content—is crucial for determining marketability and consumer appeal. Despite the increasing recognition of microbial communities' potential to influence plant health, these quality traits have often been overlooked in related studies[13]. Existing research suggests that microbial communities in the rhizosphere and on plant surfaces can influence fruit characteristics, including flavor, texture, and the plant's ability to withstand post-harvest stress[14−16]. This raises a crucial question: how microbial diversity may not only enhance disease resistance but also directly impact the quality of harvested fruit—traits that are essential for both commercial success and nutritional value.
To investigate the potential impact of microbiome diversity on fruit quality, our study focuses on two watermelon varieties, Citrullus lanatus var. vulgaris (M1511-3) and Citrullus mucosospermus (PI595203), which exhibit distinct levels of domestication. Our objective is to analyze the composition and functional dynamics of their associated microbiomes, uncovering the intricate interactions between microbial communities and fruit traits. We hypothesize that the domesticated variety, Citrullus lanatus var. vulgaris (M1511-3), may harbor a more specialized microbiome, potentially linked to key fruit quality attributes such as sweetness and tenderness. In contrast, the wild ancestor, Citrullus mucosospermus (PI595203), is expected to support a more diverse microbial ecosystem, which may enhance its ecological resilience and improve post-harvest storage potential. The observed differences in microbiome composition may reflect alterations in critical metabolic pathways that influence fruit development, stress tolerance, and overall quality. By advancing our understanding of how microbiomes shape plant traits, this study seeks to provide valuable insights for crop improvement and the promotion of sustainable agricultural practices.
-
Building on previous research, our study utilized two watermelon seed varieties with differing levels of domestication—Citrullus lanatus var. vulgaris (M1511) and Citrullus mucosospermus (PI595203), which were obtained from the laboratory. The research was conducted at the Wuwangnong Agricultural Base in Hangzhou, Zhejiang Province, China (120°12' E, 30°16' N) starting in 2022. These two diploid watermelon cultivars, which exhibit varying degrees of domestication, were grown under controlled greenhouse conditions. Over the past two years, sowing occurred once each in spring and autumn, with seeds from the previous season used for subsequent plantings. After two growing seasons, these two varieties, differing in domestication levels, were selected for further study. To ensure uniform fruit development, only one fruit per plant was retained, and manual pollination was performed at the 3rd to 5th female flower stage. Each flower was clearly marked to track fruit development.
Sampling and sample preparation for analysis
-
The sampling took place on June 15, 2023, when the watermelons reached maturity, approximately 40 d after pollination, with both cultivars maturing simultaneously.
Watermelons from two cultivars were randomly selected for analysis. For each cultivar, three fruits were randomly chosen to measure fruit sweetness and firmness. In terms of microbiome sampling, a three-point sampling method was employed to collect samples from the central flesh of each fruit, ensuring representativeness. Additionally, to provide a comprehensive analysis of the microbial communities, primary root and leaf samples were also collected from each cultivar. A total of 36 flesh samples (3 technical replicates × 3 biological replicates × 2 cultivars × 2) were collected for microbiome sequencing and metabolomics analysis. Furthermore, 18 primary root samples (3 technical replicates × 3 biological replicates × 2 cultivars) and 18 leaf samples (3 technical replicates × 3 biological replicates × 2 cultivars) were collected for microbiome sequencing analysis. During the sampling process, all tools were sterilized to prevent contamination, ensuring the reliability of the samples and providing a solid foundation for subsequent microbiome analysis[17]. All samples were immediately sterilized after collection to remove surface contaminants and rapidly frozen in liquid nitrogen to preserve microbial composition and metabolic activity. They were subsequently stored at −80 °C for further analysis. Additionally, seeds were harvested from each watermelon for phenotypic analysis[18].
Phylogenetic tree analysis of watermelon varieties
-
To construct the CDS sequences for seven watermelon varieties and build a phylogenetic tree, we employed the maximum likelihood method[19]. CDS sequences for the varieties were first obtained and analyzed for orthologous gene groups using OrthoFinder[20]. For multiple sequence alignment (MSA) of single-copy orthologs, the MAFFT tool was utilized[21]. The aligned sequences were then trimmed with trimAl to focus on conserved regions[22]. Next, the sequences were processed and formatted using seqkit to ensure proper structure for downstream analysis[23]. Finally, a phylogenetic tree was constructed using IQ-TREE 2.0, facilitating the inference of evolutionary relationships among the watermelon varieties and enabling the analysis of their sequence diversity and evolutionary patterns based on CDS data[24].
Measurement of fruit sweetness and firmness
Sweetness measurement
-
Watermelon sweetness was measured using a digital refractometer (Atago, Japan). A small juice sample was extracted from the central flesh using a sterilized knife and placed on the refractometer prism. The refractive index was recorded and reported as °Brix, representing the percentage of soluble solids, primarily sugars. Each measurement was performed in triplicate for each sample to ensure accuracy and reproducibility[25].
Firmness measurement
-
Fruit firmness was measured using a texture analyzer (Stable Micro Systems, UK) with a 2 mm cylindrical probe, which penetrated the central flesh to a depth of 20 mm at 1 mm/s. The maximum force (N) required for penetration was recorded, with measurements taken from three different locations in each fruit to ensure consistency. Each measurement was repeated in triplicate for accuracy and reproducibility[26].
Metabolomic analysis and differential metabolite identification
Metabolite extraction and quality control sample preparation
-
A 25 mg sample of watermelon flesh from each variety (three biological replicates per variety) is weighed and placed in an EP tube under low-temperature conditions. Homogenization beads, 500 μL of extraction solvent (methanol : acetonitrile : water = 2:2:1, v/v), and an isotope-labeled internal standard are added. The sample is vortexed for 30 s, homogenized at 35 Hz for 4 min, and sonicated for 5 min (repeated three times). After standing at −40 °C for 1 h, the sample is centrifuged at 12,000 rpm (13,800 × g) for 15 min at 4 °C. The supernatant is collected, and equal volumes from all samples are combined to create a quality control (QC) sample[27,28].
UHPLC-MS/MS setup for metabolomic profiling
-
Instrumental analysis is performed using a Thermo Fisher Vanquish UHPLC system with a Phenomenex Kinetex C18 column (2.1 mm × 50 mm, 2.6 μm). Mobile phase A is water with 0.01% acetic acid, and phase B is a 1:1 mixture of isopropanol and acetonitrile. The injection volume is 2 μL, with the sample tray at 4 °C. Mass spectrometry is conducted on an Orbitrap Exploris 120 with the following parameters: sheath gas flow 50 Arb, aux gas flow 15 Arb, capillary temperature 320 °C, MS resolution 60,000, MS/MS resolution 15,000, collision energy 20/30/40, and spray voltage ± 3.8 kV. Real-time monitoring ensures stable signal quality, with QC samples showing a correlation > 0.85 for high data quality[29,30].
Metabolite identification: data preprocessing and analysis
-
Data preprocessing includes removing outliers using RSD, filtering missing values (< 50%), and imputing remaining values with 'half of the minimum value'. Data is normalized with an internal standard (IS) for consistency. Differential metabolites are identified using t-tests or ANOVA and mapped to KEGG pathways to explore their biological roles[27,28,31].
Metagenomic assembly and analysis
Watermelon DNA library preparation and sequencing
-
DNA was extracted from the samples using a DNA Isolation Kit and quantified with a Qubit fluorometer[32]. Samples meeting quality standards were selected and randomly fragmented into approximately 350 bp segments using a Covaris ultrasonic processor[33]. The fragments underwent end repair, A-tailing, adapter ligation, purification, and PCR amplification to complete library preparation. The library concentration was initially quantified using a Qubit 2.0 fluorometer and diluted to 2 ng/μL[34]. Insert size was verified with an Agilent 2100 Bioanalyzer. Quantitative PCR (Q-PCR) confirmed that the effective concentration exceeded 3 nM. After quality control, libraries were pooled based on concentration and sequencing requirements and subjected to Illumina PE150 sequencing[35].
Sequencing, quality control, and data filtering
-
Following library preparation, Illumina PE150 sequencing was performed. The raw sequencing data initially contained low-quality reads, which were subsequently filtered to ensure accuracy and reliability for downstream analysis[35]. To eliminate host genomic sequences from watermelon, we used two tools: Bowtie2 and fastp. First, Bowtie2 was employed to align the sequencing data against the reference watermelon genome, allowing us to identify and remove host-specific sequences. These watermelon genomic sequences were excluded, leaving only microbial genomic data for further analysis[36]. Fastp was then employed for quality control, setting a minimum quality score of Q20 and a read length threshold of 50 bp to filter out low-quality reads and short fragments. It also removed adapter contamination and further refined the data to ensure it met the quality standards required for subsequent analysis[37]. These steps yielded microbial genomic data, free of host-derived sequences, ensuring accuracy and reliability.
De novo assembly and gene prediction
-
De novo assembly of the processed sequencing data was conducted using the MetaSPAdes tool, which generated contigs and scaffolds, excluding fragments shorter than 500 bp to ensure data integrity[38]. Gene prediction was carried out with Prodigal, producing both DNA and protein sequences, followed by length-based filtering[39]. These sequences were then clustered into non-redundant sets using CD-HIT and the corresponding protein sequences were extracted via a custom Perl script[40]. Finally, a Salmon index was constructed for gene quantification from the raw sequencing data[41].
Microbial community analysis and functional annotation
-
The HUMAnN2 tool suite was employed for multi-level functional annotation, encompassing pathway coverage and gene family identification[42]. Bowtie2 and MetaPhlAn4 were utilized for reference library construction and microbial community composition analysis[36,43]. Gene families were classified, and normalized abundance data were visualized. Species abundances were plotted using Graphlan[44], while LEfSe analysis was conducted to identify significant biomarker species that exhibited differential abundances between groups[45].
Sequence annotation and functional profiling
-
The non-redundant sequences generated by CD-HIT were subsequently annotated using the eggNOG, COG, CAZyme, and CARD databases[40,46−49]. The eggNOG database provided annotations of orthologous gene clusters and functional categories, while the COG database classified genes into functional groups[46,47]. The CAZyme database identified carbohydrate-active enzyme-related genes, and the CARD database identified antibiotic-resistance genes[48,49]. These comprehensive annotations provided insight into gene functionality and resistance profiles and enabled the comparison of functional gene differences between samples.
-
A phylogenetic tree constructed from coding sequences (CDS) during watermelon ripening reveals significant differences in domestication levels between M1511-3 and PI595203[19,50] (Fig. 1a; Supplementary Table S1). The phylogenetic tree indicates that the M1511-3 variety, belonging to Citrullus lanatus var. vulgaris, has undergone a longer evolutionary process and exhibits a higher degree of domestication. In contrast, the PI595203 variety, which belongs to Citrullus mucosospermus, shows a lower degree of domestication and is more closely related to its wild ancestors. The M1511-3 variety exhibited patterned skin and bright red flesh, while PI595203 displayed unmarked skin and pale flesh (Fig. 1b−e). In terms of sweetness and texture, M1511-3 outperforms PI595203, with a significantly higher level of sweetness (Fig. 1f; Supplementary Table S2). Additionally, the flesh of PI595203 is much firmer, being up to ten times harder than that of M1511-3 (Fig. 1g; Supplementary Table S2). These observations highlight the marked differences in quality traits between the two cultivars, with the domesticated M1511-3 excelling in sweetness and appearance, but exhibiting a softer flesh. Further analysis reveals that the microbial composition in the leaves and roots shows relatively minor differences after the fruit ripens (Fig. 1h, i; Supplementary Table S2), suggesting stability in the microbial communities of these tissues. In contrast, a significant increase in microbial diversity is observed in the flesh after ripening (Fig. 1j; Supplementary Table S3), indicating that the microbial communities in the flesh become more diverse as the fruit matures. This suggests that while the microbial ecology in the mature flesh becomes richer, the microbial composition in the leaves and roots remains relatively consistent.
Figure 1.
Differences in fruit characteristics and microbial communities among watermelon cultivars. (a) Phylogenetic tree of watermelon and related Cucurbitaceae species. (b) Fruit appearance of M1511-3. (c) Fruit section of M1511-3. (d) Fruit appearance of PI595203. (e) Fruit section of PI595203. (f) Differences in flesh firmness. (g) Differences in flesh sweetness. (h) Differences in microbial communities across leaves, roots, and flesh.
Functional profiling of metabolic divergence in watermelon varieties: implications for fruit quality
-
To explore the differences in fruit quality between two watermelon varieties, we systematically identified a range of metabolites exhibiting significant variation in their abundance. By mapping these metabolites to their respective metabolic pathways using the KEGG database, we uncovered pronounced discrepancies in both the relative abundance and expression levels of metabolites in the mature flesh of the two varieties. The less domesticated variety, PI595203, exhibited elevated expression levels in nucleotide metabolism and D-amino acid metabolism pathways, implying that it may have evolved distinct metabolic adaptations to better withstand environmental stressors. In contrast, the more domesticated variety, M1511-3, demonstrated heightened metabolic activity in pathways linked to carbohydrate metabolism, amino acid metabolism, lipid metabolism, and transport and translation. This suggests that M1511-3 harbors a more intricate and refined metabolic profile, reflecting its optimization of growth and metabolic processes. Consequently, M1511-3 is likely to exhibit superior fruit quality traits, including enhanced sweetness and improved texture. Further analysis of the differential metabolites revealed a significant upregulation of several KEGG pathways in M1511-3 (Fig. 2b; Supplementary Table S4). Notably, pathways related to ABC transporters ('ko02010') and amino acid biosynthesis ('ko01230') were markedly upregulated. The upregulation of the 'ko02010' pathway signifies an enhanced efficiency in molecular transport, which may facilitate improved nutrient uptake and distribution, thereby supporting fruit growth and enhancing quality. Furthermore, the upregulation of the 'ko01230' pathway suggests an augmented capacity for amino acid biosynthesis, a crucial process for plant growth, metabolic function, and the development of fruit flavor and nutritional value.
Figure 2.
Analysis of KEGG pathway enrichment and gene expression differences. (a) KEGG enrichment highlights significant KO categories in cultivated (pink) and wild (blue) watermelon flesh. (b) KEGG plot shows enriched pathways in M1511-3 vs PI595203.
Domestication drives microbial diversity loss and alters community composition in watermelon flesh
-
In conclusion, the analysis of microbial communities in the flesh of Citrullus lanatus var. vulgaris M1511-3 and Citrullus mucosospermus PI595203 reveals notable differences in microbial composition and diversity between the two cultivars (Fig. 3a, b). Both cultivars predominantly harbor microbes from the Proteobacteria phylum, including Alphaproteobacteria and Gammaproteobacteria. Specifically, the microbial community in the flesh of Citrullus lanatus var. vulgaris M1511-3 is consistently dominated by Proteobacteria, with a significantly higher abundance of Alphaproteobacteria compared to Gammaproteobacteria. This pattern is in contrast to that observed in Citrullus mucosospermus PI595203. Within the Alphaproteobacteria, the abundance of Sphingomonadales is relatively high across all replicates, with the representative species Sphingomonas maintaining a stable abundance (approximately 69.69% to 89.26%), which is significantly higher than in PI595203. In contrast, the microbial community in the pulp of Citrullus mucosospermus PI595203 (Fig. 3c) is also dominated by Proteobacteria, with an abundance ranging from 69.66% to 81.82%. However, PI595203 also hosts a more diverse microbial community, in particular with a higher presence of Firmicutes, mainly represented by Bacilli, which account for 14.60% to 26.02% of the total community. In addition, Actinomycetia are present at relatively low levels (0.33% to 5.57%), along with other minor microbial taxa (Fig. 3e; Supplementary Tables S5, S6).
Figure 3.
Comparative analysis of microbial communities in two cultivars. (a) LDA plot showing species with values > 2, denoted by D for M1511-3 and W for PI595203. (b) Evolutionary plot depicting taxonomic levels and biomarkers. (c), (d) Phylogenetic trees of microbial communities in both cultivars. (e) Abundance differences of key microbial species between the two varieties.
Differential microbial functional profiles between domesticated and wild watermelon cultivars
-
Based on the clustering of orthologous genes (COG) from the assembled genomes, we identified significant differences in the functional categories of the fruit microbiomes between the two watermelon cultivars (Fig. 4a). The microbiome of the highly domesticated variety M1511-3 exhibited significantly higher expression levels in categories such as 'Cellular Processes and Signal Transduction' and 'Metabolism'. In contrast, the microbiome of the PI595203 variety showed higher expression in categories related to inorganic ion transport, amino acid transport, and intracellular transport. These differences may influence the overall resilience and fruit quality of each cultivar, underscoring the importance of microbial dynamics in agricultural practices (Supplementary Table S7).
Figure 4.
Functional Differences in Watermelon Flesh Microbiota: (a) COG annotation of microbiota (Group D: M1511-3, Group W: PI595203; n=3 replicates); (b) KEGG pathway enrichment (left: raw data, right: secondary classification); (c) CAZyme family abundance differences, highlighting enrichment in (d) M1511-3 and (e) PI595203 microbiota; (f) 40 AMR genes detected in PI595203, annotated by localization, family, and resistance mechanisms.
According to the KEGG pathway enrichment results, significant differences in metabolic activity were observed between the two watermelon cultivars (Fig. 4b). The microbiome of M1511-3 exhibited higher metabolic activity in energy metabolism, carbohydrate metabolism, and the biosynthesis of other secondary metabolites. In contrast, the microbiome of PI595203 showed almost no metabolic activity in energy metabolism. In terms of signaling and cellular processes, M1511-3 exhibited higher expression of genes related to intercellular signaling and cellular movement, while PI595203 showed a similar trend. Additionally, the microbiome of PI595203 displayed increased activity in pathways related to disease and immune responses, indicating stronger resistance to pathogens and antibacterial pressures. These differences highlight the impact of domestication on microbial dynamics, influencing the adaptability and resilience of each cultivar (Supplementary Table S8).
The CAZyme annotation results revealed significant differences in the types and abundances of carbohydrate-active enzymes (CAZymes) between the microbial communities of the two watermelon cultivars (Fig. 4c). Specifically, the microbiome of the highly domesticated M1511-3 cultivar exhibited higher enzyme activity in certain CAZyme families, such as AA5 and CBM50, indicating greater potential for redox reactions and carbohydrate-binding (Fig. 4d). However, in other families, particularly glycoside hydrolases (GHs) and carbohydrate-binding modules (CBMs), enzyme activity in M1511-3 was significantly lower, with certain GH families, such as GH0 and GH3, showing almost undetectable activity. In contrast, the microbiome of the less domesticated PI595203 cultivar showed significantly higher activity in several glycoside hydrolase (GH), carbohydrate-binding module (CBM), carbohydrate esterase (CE), and glycosyltransferase (GT) families, particularly in GH1, GH10, GH13, GH43, GT1, and GT4. This suggests that the microbiome of this wild cultivar retains a broader range of enzymatic activities, enabling it to efficiently utilize various carbohydrates and adapt to environmental changes. In comparison, PI595203 showed no activity in redox enzymes and certain carbohydrate-binding modules, likely reflecting its ecological requirements in a natural growth environment (Supplementary Table S9).
CARD annotation of the microbial community in the flesh of the less domesticated watermelon cultivar PI595203 identified approximately 40 antibiotic resistance genes, which function through various mechanisms such as reduced antibiotic permeability, antibiotic inactivation, target protection, target modification, and antibiotic efflux (Fig. 4f). Notably, antibiotic efflux pumps represent a primary resistance mechanism, enabling bacteria to actively expel antibiotics and prevent them from reaching their targets. Several genes associated with antibiotic efflux, including mef(F), YojI, mdtA, mdtB, mdtC, emrB, mdtE, mdtF, cpxA, KpnG, msbA, and tet(B), showed high sequence similarity, typically ranging from 99% to 100%. Genes such as mdtE, mdtF, and emrB displayed perfect sequence alignment, indicating their high conservation within this species. In contrast, no antibiotic resistance genes were detected in the microbial community of the more domesticated cultivar M1511-3 (Citrullus lanatus var. vulgaris) (Supplementary Tables S10).
-
Domestication is a crucial process that not only shapes the genetic characteristics of crops but also profoundly alters the microbial communities with which they interact[51]. Our study aims to investigate the disparities in internal microbial communities, metabolic activities, and fruit quality traits between Citrullus lanatus var. vulgaris (M1511-3) and its wild relative, Citrullus mucosospermus (PI595203), to elucidate their adaptability and evolutionary trajectories under divergent ecological conditions. Our results reveal marked differences in microbial community composition, metabolic activity, and fruit quality between M1511-3 and PI595203, which are closely associated with their growth performance and stress resilience. As a cultivated variety, M1511-3 exhibits increased fruit sweetness, underpinned by a microbial community predominantly composed of Alphaproteobacteria, notably the Sphingomonas genus, which plays a pivotal role in promoting fruit development and sweetness. In contrast, PI595203 harbors a more complex and diverse microbial community, comprising not only Alphaproteobacteria but also an abundance of Gammaproteobacteria, including Enterobacterales and Pseudomonas species, indicative of enhanced ecological adaptability and disease resistance. Our findings align with previous studies on crop domestication and its impact on microbial communities. It is well-established that domestication often leads to a reduction in microbial diversity, which may, in turn, influence plant immunity, disease resistance, and ecological adaptability[52−55]. For example, studies on maize (Zea mays) and wheat (Triticum aestivum) have demonstrated that, while core microbial communities remain stable, domestication significantly alters the abundance and diversity of root-associated microbes[56,57]. This reduction in microbial diversity is often linked to diminished stress tolerance and pathogen resistance, ultimately impacting plant health and yield. In contrast, wild relatives typically retain higher microbial diversity, which enhances disease resistance and adaptability to challenging environmental conditions. In our study, the diverse microbial community observed in PI595203 is consistent with these findings, suggesting enhanced ecological adaptability in natural environments[58]. The diverse microbial community observed in PI595203 further reinforces these findings, highlighting the exceptional adaptability of wild species to their natural environments.
Further analysis suggests that the abundance of Sphingomonas species in M1511-3 is likely intricately linked to their ability to promote plant growth and fruit development. Previous studies have established that Sphingomonas bacteria synthesize plant hormones such as gibberellins (GA) and indole-3-acetic acid (IAA), which are essential for the growth of roots, stems, and leaves, as well as for the early stages of fruit development[7,59]. Through hormonal regulation, M1511-3 experiences accelerated growth, particularly under environmental stress, where Sphingomonas plays a pivotal role in activating the plant's immune response. This activation not only aids in the plant's adaptation to adverse conditions but also contributes to the enhanced sweetness and flavor of the fruit[60−62]. In contrast, the microbial community of PI595203 not only contains beneficial Sphingomonas bacteria but also harbors a diverse array of other microorganisms, including potential pathogens such as Dickeya aquatica (from the order Enterobacterales) and Pseudomonas syringae (from the genus Pseudomonas)[63−65]. The presence of these pathogens suggests that PI595203 must contend with harmful microorganisms in its natural environment, likely prompting the plant to activate adaptive mechanisms to mitigate pathogen pressure. Concurrently, beneficial microbes, such as those from the Bacillus genus (belonging to the Bacilli class), secrete hydrolytic enzymes that inhibit pathogen growth, thereby enhancing the plant's resistance to both diseases and environmental stresses[66−69]. Additionally, the microbial community of PI595203 comprises a small proportion of Actinomycetia, which belong to the phylum Actinobacteria and are renowned for their production of antibiotics, such as streptomycin. These microorganisms play a crucial role in pathogen suppression, further contributing to the plant's defense mechanisms[70]. Notably, Streptomyces species act as potent antimicrobial and antifungal agents, effectively inhibiting the growth of a broad spectrum of root-borne pathogens[4]. Moreover, these microbes facilitate nutrient uptake, thereby enhancing the plant's overall health and vitality. Together, the dual functions of these beneficial microorganisms underscore the remarkable ecological adaptability and resilience of PI595203 in managing both pathogen threats and nutrient dynamics.
In addition to differences in microbial community composition, variations in metabolic activity further emphasize the adaptive characteristics of the two cultivars. The heightened expression of key pathways, such as nucleotide and D-amino acid metabolism, in PI595203 indicates a robust metabolic regulatory capacity, enabling the plant to effectively cope with environmental changes under resource-limited or stressed conditions[71]. In contrast, M1511-3 exhibits optimized pathways in carbohydrate, amino acid, and lipid metabolism, as well as in transport and translation processes. These metabolic adjustments not only support the plant's growth and development but also enhance the sweetness, flavor, and nutritional value of its fruit[72−75]. The efficiency of nutrient uptake and transport in M1511-3 contributes to the enhanced accumulation of nutrients in the fruit, thereby improving its taste and flavor.
The functional analysis of the microbial communities further reveals that the microbiome of M1511-3 shows higher expression levels in categories such as 'cellular processes and signal transduction' and 'metabolism'. These enhanced functions support its superior adaptation to cultivated environments and contribute to the production of high-quality fruit[76,77]. In contrast, the microbiome of PI595203 exhibits heightened activity in categories related to inorganic ion transport, amino acid transport, and intracellular transport. These traits reflect its adaptive advantages in responding to resource limitations and environmental stress under natural conditions[78,79]. In the enzyme activity analysis within the CAZyme (carbohydrate-active enzyme) families, the microbial community of M1511-3 exhibited elevated enzyme activities in families such as Auxiliary Activity Family 5 (AA5) and Carbohydrate-Binding Module Family 50 (CBM50). This indicates an enhanced capacity for redox reactions and carbohydrate binding, further supporting its metabolic versatility[80,81]. However, enzyme activities in other glycoside hydrolase (GH) and carbohydrate-binding module (CBM) families were significantly lower, suggesting that M1511-3 may optimize fruit quality and nutritional composition through alternative metabolic pathways[82,83]. In contrast, the microbial community of PI595203 exhibited a broader spectrum of enzyme activities, particularly across multiple glycoside hydrolase (GH), carbohydrate-binding module (CBM), carbohydrate esterase (CE), and glycosyltransferase (GT) families. This extensive enzymatic profile suggests that PI595203 is more efficient in utilizing a diverse range of carbohydrates, likely enhancing its ability to adapt to complex environmental conditions[82−85]. Moreover, the microbial community of PI595203 harbors approximately 40 antibiotic-resistance genes, which confer protection against antibiotic stress through various mechanisms. These include reduced antibiotic permeability, antibiotic inactivation, target protection, target modification, and antibiotic efflux. Efflux pumps, the primary mechanism of resistance, actively expel antibiotics from bacterial cells, thereby preventing their action on target bacteria, such as Pseudomonas[86−88]. In contrast, no antibiotic resistance genes were detected in the microbial community of M1511-3, suggesting that its microbial community relies more on optimized metabolic pathways and regulatory mechanisms to enhance fruit quality, rather than on antibiotic resistance mechanisms.
Our study highlights key insights into microbial community composition and metabolic activity but leaves microbial-plant interactions largely unexplored. Future research using metagenomics and functional genomics could reveal how specific microbes influence plant growth, immunity, stress tolerance, and fruit quality. Despite these gaps, the findings offer practical value for agriculture. Introducing beneficial microbes like Sphingomonas may enhance the resilience and fruit quality of wild varieties, while diversifying microbial communities in cultivated varieties like M1511-3 could improve disease resistance and adaptability. Metabolic pathway optimization, such as boosting disease resistance in PI595203 or enhancing nutrient transport in M1511-3, could further improve yield and nutritional value. These insights provide a foundation for advancing agricultural biotechnology and sustainability.
-
Our study explores the impact of domestication on microbial communities and their subsequent effects on metabolic activity and fruit quality in two watermelon cultivars: the domesticated Citrullus lanatus var. vulgaris (M1511-3) and its wild relative, Citrullus mucosospermus (PI595203). While the role of microbial communities in plant health, disease resistance, and stress tolerance is well-established, their specific influence on fruit quality remains underexplored. Our findings reveal that domestication significantly alters microbial composition, which in turn plays a pivotal role in plant growth, fruit development, and quality traits. M1511-3, the domesticated cultivar, harbors a specialized microbial community predominantly composed of Sphingomonas bacteria, which contribute to the enhanced sweetness and development of the fruit. In contrast, PI595203 maintains a more diverse microbial ecosystem, including Gammaproteobacteria, Bacilli, and Actinomycetia, which bolster its ecological adaptability and pathogen resistance. These differences in microbial composition are closely associated with metabolic variations: PI595203 demonstrates superior stress tolerance, while M1511-3 exhibits optimized metabolic pathways that enhance fruit quality, including sweetness, texture, and nutritional value. Our research highlights the essential role of microbial communities in both disease resistance and fruit quality development. Our findings provide key insights into sustainable agricultural practices, emphasizing the importance of microbial diversity in improving crop resilience, yield, and quality, while contributing to the advancement of agricultural biotechnology.
The authors thank Mr. Yazhou Wang for assist of bioinformatic analysis. This study was partially supported by a grant from the National Natural Science Foundation of Hainan Province (325CXTD616), and Hainan Seed Industry Laboratory and China National Seed Group (Project of B24YQ0002).
-
The authors confirm contribution to the paper as follows: study conception and design: Yang J; Y.L. performing most of the experiments and data analysis: Liu Y; experiment and data analysis assisting: Yanf Y, Zhang X; draft manuscript preparation: Liu Y; manuscript revision: Yang J, Hu Z, Zhang M. All authors reviewed the results and approved the final version of the manuscript.
-
The datasets generated during and/or analyzed during the current study are available from the corresponding author on reasonable request.
-
The authors declare that they have no conflict of interest.
- Supplementary Table S1 Phylogenetic tree of seven watermelon species based on single-copy genes.
- Supplementary Table S2 Comprehensive fruit quality data for two watermelon cultivars, including firmness and sweetness measurement.
- Supplementary Table S3 Microbial abundance data in theleaves, roots, and flesh of two watermelon cultivar.
- Supplementary Table S4 KEGG pathway annotation data for the pulp of two watermelon cultivars.
- Supplementary Table S5 Microbial abundance data across different plant tissues.
- Supplementary Table S6 Linear discriminant analysis (LDA) comparing microbial communities in the flesh of two watermelon cultivars (significant LDA values 40).
- Supplementary Table S7 COG annotation results for the microbial community in the flesh of two watermelon cultivars.
- Supplementary Table S8 KEGG pathway annotation results for the microbial community in the flesh of two watermelon cultivars.
- Supplementary Table S9 Table S9 CAZyme annotation results for the microbial community in the flesh of two watermelon cultivars.
- Supplementary Table S10 CARD enrichment analysis for antibiotic resistance genes.
- Copyright: © 2025 by the author(s). Published by Maximum Academic Press, Fayetteville, GA. This article is an open access article distributed under Creative Commons Attribution License (CC BY 4.0), visit https://creativecommons.org/licenses/by/4.0/.
-
About this article
Cite this article
Liu Y, Yang Y, Zhang X, Hu Z, Zhang M, et al. 2025. Microbiome analysis uncovers fruit-specific microbial community divergence between wild and cultivated watermelon varieties. Vegetable Research 5: e011 doi: 10.48130/vegres-0025-0006
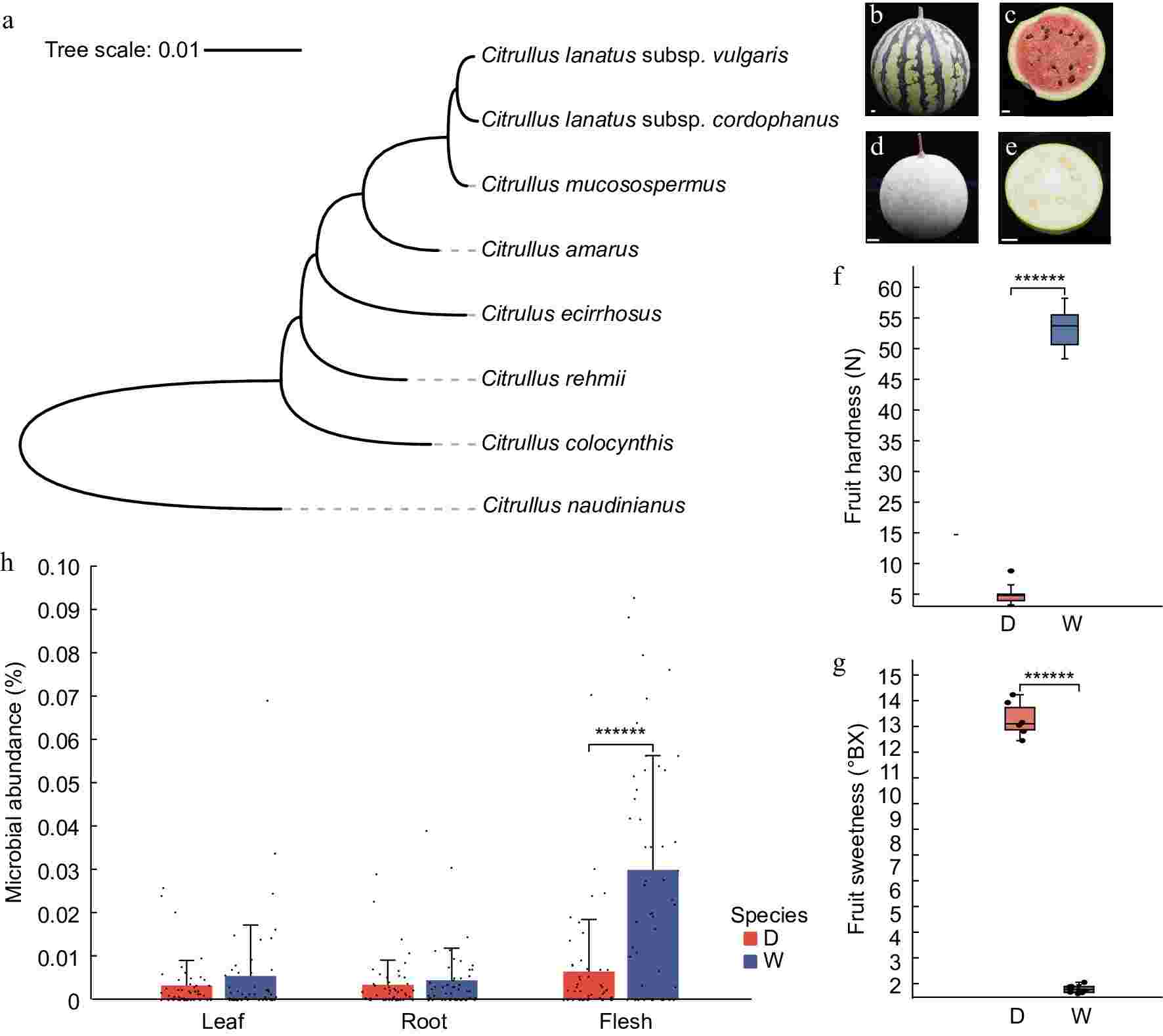